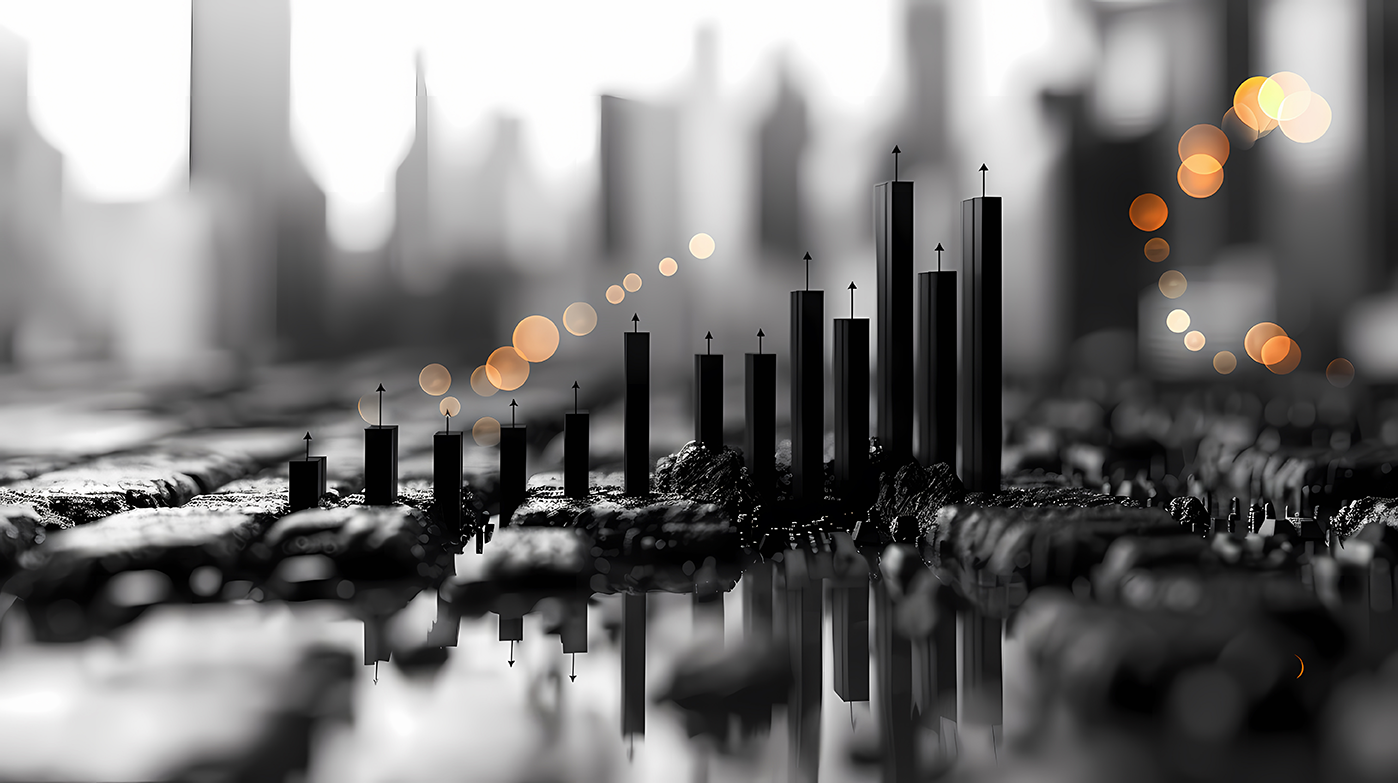
In today’s digital landscape, the success of many businesses hinges on their ability to effectively prioritize data projects and products. As companies navigate this landscape, they face the challenge of balancing expected value generation against costs, all while evaluating the merits of individual projects versus holistic product development. In this article, we’ll delve into the intricacies of portfolio management within the realm of data, exploring various methodologies, addressing challenges, and presenting strategies for success.
Portfolio Management: A Systemic Approach
At the core of effective portfolio management lies a rigorous process of weighing various projects against each other. In many organizations, different divisions often vie for resources and attention, making it imperative to employ a value-based judgment linked to risk and investment costs. Our clients prefer to use value-based judgment linked to risk and investment costs, usually based on turnover, margins and opportunity costs. However, when evaluating data products, a standardized approach is crucial. This involves considering factors such as project scale, duration, and legal implications to ensure alignment with overarching corporate strategies.
Challenges in Data Project and Product Development
From a financial perspective, data products operate akin to conventional products, necessitating an initial investment encompassing the costs of the data project itself, along with subsequent maintenance and operational expenses.
Setting up the data platform and assembling the data team routinely grapples with inadequate representation and funding. Conversely, individual use cases often operate independently, leveraging existing resources. Portfolio management faces a dilemma: while everyone desires recognition for the self-sufficient use cases, reluctance persists in investing in the foundational data team and platform.
The crux of challenges in data projects and product development lies in data quality. While building upon established and secure data is preferable, it’s seldom the reality within a company. This underscores the second significant obstacle: a universal desire for secure, high-quality data, juxtaposed with reluctance to invest in achieving it. Regrettably, the significance of data quality is often perceived as somewhat abstract. There’s a pervasive belief that it can be addressed swiftly or “smartly”, relying on minimal effort and the right methodology. However, such promises from product manufacturers and management consultants often fall short of reality.
Each data integration project is distinctive and demands a unique approach. The labor involved in comprehending the data and its specifications typically outweighs the implementation phase. Furthermore, the data refinement process, encompassing integration, testing, deployment, and stabilization, almost invariably surpasses the timeline of the meticulously planned initial stages of the project.
Prioritization Frameworks: Finding the Right Fit
Various standards or frameworks exist in the market for prioritizing projects and products, which assess business requirements based on their value contribution and associated costs. When comparing potential project candidates in terms of feasibility, a ratio of one-fifth to one-half feasibility is commonly encountered in corporate settings. However, this ratio and assertion warrant careful consideration as they often overlook the maturity level of the data product or project.
Prioritization frameworks or guidelines become increasingly crucial as demand escalates. The Weighted Shortest Job First (WSJF) serves as a solid foundation here, considering the expected value contribution relative to the effort expended, while also factoring in runtime considerations. This method has gained prominence, particularly through its integration with SaFe (Scaled Agile Framework). Notably, WSJF divides the Cost of Delay by Job Duration, encompassing not only monetary value but also time sensitivity and risk factors. Emphasizing time-to-market, the method aligns closely with product development strategies.
Yet, does WSJF address all considerations and remain universally applicable? Is it conceivable that WSJF operates solely at the backlog level? These questions prompt further examination of the method’s scope and applicability across diverse contexts.
Managing Expectations and Cultivating Teams- limits of prioritization frameworks
As previously stated, data products often lack the clearly defined state required for conventional methods. The Weighted Shortest Job First (WSJF) method, which emphasizes swift value addition, tends to favor product features with rapid impact.
However, rigid adherence to WSJF could result in prioritizing only small, easily quantifiable features, inevitably sidelining new initiatives. This inclination towards small, well-defined features, driven by the typical involvement of key stakeholders, risks neglecting emerging topics. To prevent this, there’s a pressing need to explicitly endorse numerous small feasibility studies and discovery tasks. Failure to do so would subject new endeavors to overly stringent prioritization, hindering their progress.
Moreover, the prioritization strategy outlined above tends to accumulate technical debt and compromise data integrity over time. To address this, embracing broader concepts and adopting a different mindset becomes imperative. This shift opens avenues for innovation and ensures a more balanced approach to product development.
The Phased Approach: Iterative Progress
Before embarking on a data project, it’s common to encounter a lack of maturity either in the project itself or in the initial stages of product development. When new product analyses or data are absent, prioritization frameworks become insufficient for evaluation. This immaturity in prioritization poses multifaceted challenges, highlighting the critical need for management involvement: clarifying requirements, understanding user needs, and identifying customers. Securing sponsorship and budget are also frequent hurdles. Moreover, the absence of a defined framework for initiating new projects with data product characteristics due to a lack of experience exacerbates the situation. Unclear responsibilities and the absence of preliminary analyses and architectural specifications further complicate matters.
Data products with extensive and varied databases offer significant benefits to customers and users, yet they pose substantial challenges due to widely distributed ownership across the organization. In this landscape, product managers and product owners assume pivotal roles. Without these roles being filled, there are no advocates to catalyze overcoming the initial significant hurdles, of which there are many. Data quality emerges as a central concern here.
The issue of poor data quality often eludes outsiders and may seem trivial. However, it extends beyond mere faulty data records to cases where data linkage is compromised, duplicates arise, or data arrives late. From an end user’s perspective, these issues constitute faults and errors. Achieving sustainable improvements in data quality demands considerable effort, dedication, and commitment. This effort may impact numerous stakeholders, as well as supply chains or the IoT enablement of end devices, a process that may unfold over years following extensive rollouts. The realm of possibilities is vast, with nothing set in stone. The development of a data product truly commences only once the data has been thoroughly assessed.
Before the project begins, the main task is to manage expectations. However, in the absence of a cohesive team and control mechanisms, setting a direction becomes imperative. While technology and methodologies have made significant strides in recent years, and platforms have improved substantially, addressing data quality remains a labor-intensive and inherently challenging endeavor. Despite considerable technological support and evolutionary progress, revolutionary developments are scarce, given the inherent complexities involved.
Cultivating a Data-Oriented Culture
To effectively manage data projects or a project portfolio, it’s crucial to address expectations upfront and the trick is to counteract, intervene and correct. This involves thorough analysis, effort estimation, architectural planning and careful management of data projects. Many data projects transition into product development, making expectation management a critical aspect.
However, numerous projects veer off course, leading to persistent backlogs despite multiple production cycles. Project managers must navigate these challenges by balancing objectives, budget constraints, and ongoing task influx. Issues such as unprepared environments, data complications, and platform limitations often hinder project progress. As interim managers, our focus is on fostering accountability, team cohesion, and establishing robust project and portfolio management structures.
In the medium term, attention shifts to refining team structures, closing gaps, and implementing frameworks for data and AI management. DevOps/MLOps practices are then employed to streamline platform enablement and ensure ongoing data integrity.
The Phased Approach: Iterative Progress
The phased approach is indispensable for many products, especially when detailed requirements cannot be fully known from the outset. Whether dealing with external or internal customers, feedback is essential for refining product features and ensuring usability. Organizations must cultivate data-driven decision-making, a process that necessitates time and experience with various data facets. In corporate environments, a phased approach typically proves more practical as it allows for comprehensive exploration of data complexities.
Delivering a Proof of Concept (POC) swiftly or expediting the go-live process is crucial for eliciting necessary feedback to derive requirements. It’s unrealistic to expect product owners or managers to define intricate requirements for complex or unique products without firsthand customer feedback.
As an interim manager, I’ve observed how companies, irrespective of budget size, are gravitating toward data orientation. While larger firms may allocate substantial budgets, smaller companies achieve comparable results and often experience a rapid advancement in data product development.
Cultivating a Data-Orientated Culture
In the realm of data projects, both successes and failures offer invaluable learning opportunities. While we’ve delved into the pitfalls extensively, individuals and organizations must experience this firsthand to truly grasp its significance.
Leading by example is essential; we can guide employees in navigating these challenges but cannot dictate their responses. Moreover, fostering team development takes precedence. Large projects and products are a collaborative effort, reliant on individuals who complement, trust, and support one another. Continuous team development is pivotal, requiring encouragement, promotion, and support.
Ultimately, success hinges on meeting real customer needs, underscoring the importance of prioritizing data projects accordingly. However, it’s the collective effort and progress of the team and the data product that truly matter. Both the organization and individual employees must embrace a mindset conducive to learning and adaptability, reflecting the overarching strategy of the company.