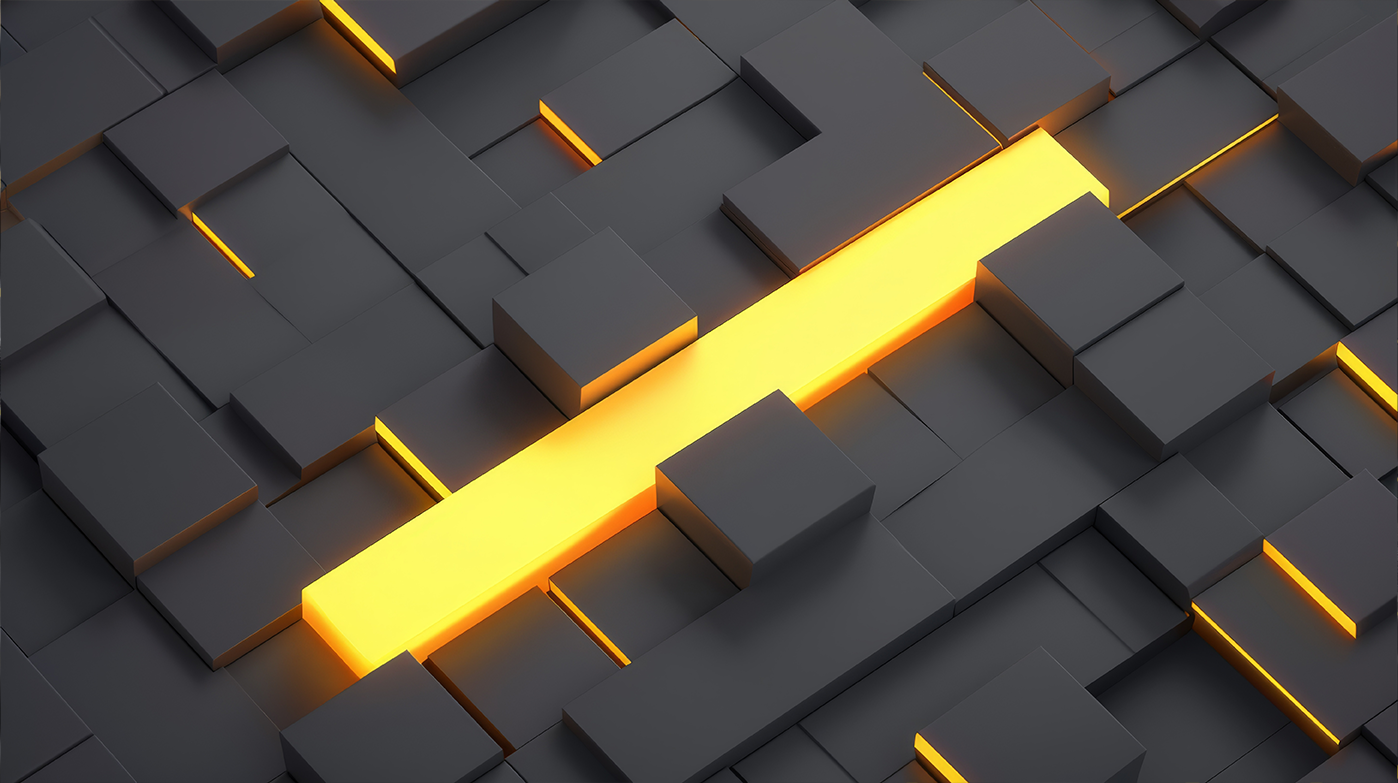
The answer is simple: By focusing on the data product, however, implementation poses a different problem entirely. Afterall, data product implementation takes place in a complex environment consisting of changing markets, companies, and many stakeholders with different objectives.
Customer-centric Approach for Data Products
Let’s first take a look at the end customer: It seems almost easy to find an existing product whose data is suitable for monetization. The creation of data products enriched with data, while conceptually promising, poses formidable challenges in terms of execution. However, such endeavors typically yield substantial value for the buyer (i.e. the end customer), manifesting as process optimization or heightened internal productivity, transcending industry boundaries.
The mere availability of additional data services can influence purchasing decisions. Consequently, companies may find themselves compelled to augment their products with data, spurred by market competitors, leading to the nuanced consideration of whether to replicate or differentiate the competitor’s product. However, product differentiation through data is worth a separate article and is therefore not the central topic here.
Digitalization and Data Availability
A pivotal consideration in the pursuit for successful data products lies in what prerequisites are needed for a ‘good’ data product. The accelerating digitalization of processes means that this data is increasingly being generated, prompting companies to make strategic decisions regarding the incorporation of data into their products. In the complex business-to-business (B2B) environment, withholding data access from customers becomes increasingly impractical. Hence, the central question shifts from whether a company offers data products to the extent of integration of data into its products.
Data Quality as a Fundamental Factor
All data is not created equal; it exists in various resolutions, temporal dimensions, and levels of quality. Data inaccuracies or poor quality impede or delay data product development. How then, can high data quality be achieved? Well, above all you need staying power. High data quality is not the result of individual measures, instead it demands sustained effort, involving extensive processes and time. While data platforms and effective data governance are indispensable for maturing data, a company’s orientation towards maintaining high data quality is equally critical. Suboptimal data quality can result in poorly differentiated data products or, worse, damage to the company’s reputation.
Product Management and Data Products
Product management assumes a pivotal role, especially when confronted with subpar data products. Convincing customers of the product’s merits becomes an arduous task when it fails to compete with rivals in aspects such as detailed usage data or IoT capability. A comprehensive view of a product extends beyond development, marketing, sales, and service to include investment in data capabilities.
Specification of Data Products
Despite advancements in data platforms, cloud technologies, and streamlined process models, companies often struggle to specify their data products. This is especially true for companies without a focus on IT. The specification of data products requires meticulous attention to detail, a step which is frequently overlooked in the pursuit of short-term success. Inadequate understanding of the task leads to ad-hoc fixes rather than a feature-driven development approach. Product owners are generally overloaded and often have to hand over the specification to developers who map the technical requirements “freestyle” in the code. The lack of understanding of the task leads to fixes and patches instead of feature-driven development.
Interdisciplinary Collaboration for Success
Successful data products emerge from collaborative efforts of diverse skill sets within teams. Knowledge of the relevant domain, facilitated by progressive data mesh approaches, underscores the importance of product orientation, interdisciplinary collaboration, and close cooperation among participants. Effective coordination by product management, prioritizing requirements through sales, becomes paramount. The trick is to achieve this cooperation between the right specialist departments and, if necessary, suppliers, data engineers and data scientists. This is controlled at a higher level by product management, which defines and prioritizes the relevant requirements through sales.
Modern Technologies and Data Platforms
The adoption of modern data platforms, particularly cloud-based, big data-driven, scalable, and real-time capable solutions, plays a crucial role in achieving success. A few years ago, only selected data scientists were able to find the right model for which the right platform was built in the BI department, one for almost every use case. At least the basic technology for this is now available off the shelf. The exceptional capabilities of artificial intelligence (AI) within these platforms have become more accessible, contributing to a paradigm shift in the landscape.
Product Development vs. IT Project Management
While technology is often readily available, the real challenge lies not in the technical area, but in a company’s mindset. Treating data products deterministically like traditional IT projects is fundamentally flawed. Above all, there are many aspects over which you have little influence, such as data from a company’s supply chain or IoT end devices in the market. In addition, you often work in an environment with too few resources during the set-up phase. The people involved lack time and expertise, the hardware lacks performance and the management lacks patience. However, a data product is not built in one piece, but in iterations. New product features tend to arise from the needs of the market.
Mindset and Cultural Change in Companies
Success in developing data products requires assembling individuals with focus, expertise, and a good dose ofpassion to define, specify, and implement products while maintaining a continuous focus during iterative development. This means nothing less than a cultural change in a company wherein teams are structured around data products. Overcoming challenges such as technological inexperience or a lack of market orientation requires an understanding of customer needs, appreciation for the importance and structure of data products, and the courage to organize teams around the product.
Our service is working on the data product, on the definition, control and implementation, above all with passion and experience.